
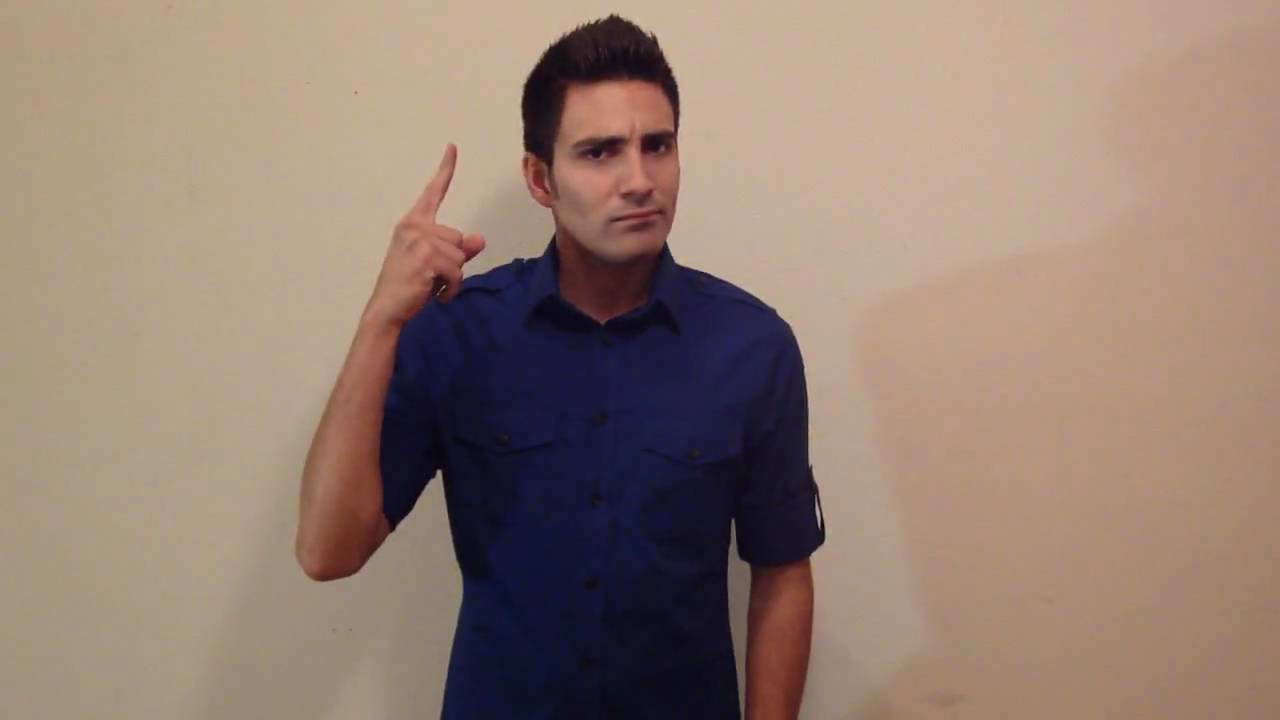
Typically, sign languages have no standardized written form. Sign languages all over the world are independent, fully fledged languages with their own grammar and word inventory, distinct from the related spoken language. Combined, the duration of the video samples is just over 24 hours long.įigure 1: Histogram of frame numbers for ASL1000 video samples. Despite that, the histogram looks like a Poisson distribution with the average of 60.
UNDERSTAND ASL SOFTWARE
There are unusual peaks for multiples of 10 frames which seems to be caused by video editing software cutting and adding captions, which favors such duration.
UNDERSTAND ASL MANUAL
After the touch-up, almost all the samples have less than 200 and more than 15 frames.įigure 1 illustrates a histogram of the duration of the 25,513 video samples of signs after the manual touch-up. In this way, around 25% of the data set was manually reviewed. There are few samples outside of the defined criteria which also have been reviewed by our annotators. We also decided to review video samples shorter than 20 frames. Although our annotators were not native in ASL, they could easily trim these video samples while considering other samples of the same label. For higher accuracy on the test set, we chose the threshold to be 6 seconds there. Therefore, we decided to manually trim all video samples with a duration of more than 8 seconds. We make the following contributions with this work:Īlthough many of the samples videos are good for training purposes, some of them include the instruction to the sign or several repeated performances with long pause in between. The sole use of RGB for sign language detection is not new but the lack of realistic large-scale data set prevent recent computer vision trends in this field.Īs such, our goal is to advance the sign language recognition community and the related state-of-the-art by releasing a new data set, establishing thorough baselines and carrying over recent computer vision trends.
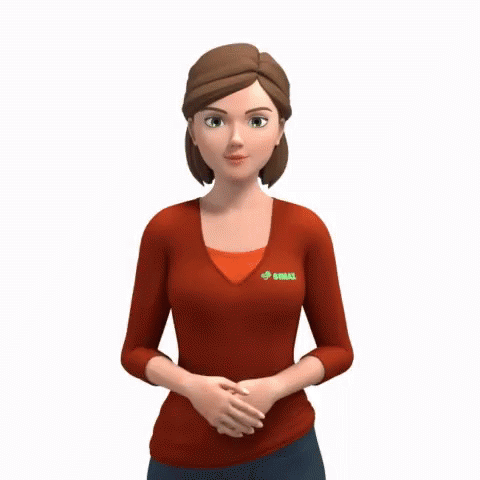

We want to support sign recognition using only a single RGB camera as we believe this will allow to design tools for general usage to empower everybody to communicate with a deaf person using ASL. However, these requirements limit the applicability to specific settings where such resources are available. Rely on external devices such as additional RGB or depth cameras, sensor or colored gloves. 1 Introductionįor decades, researcher from different fields have tried to solve the challenging problem of sign language recognition. Finally, We estimate the effect of number of classes and number of training samples on the recognition accuracy. We also propose new pre-trained model more appropriate for sign language recognition. We propose I3D, known from video classifications, as a powerful and suitable architecture for sign language recognition. We evaluate baselines from action recognition techniques on the data set. In this paper, we propose the first large scale American sign language (ASL) data set that covers over 200 signers, signer independent sets, challenging and unconstrained recording conditions and a large class count of 1000 signs. This is not a new approach but the lack of realistic large-scale data set prevented recent computer vision trends on video classification in this filed. Unlike other frequent works focusing on multiple camera, depth camera, electrical glove or visual gloves, we focused on the sole use of RGB which allows everybody to communicate with a deaf individual through their personal devices. One such challenge that we have tackled in this paper is providing accessibility for deaf individual by providing means of communication with others with the aid of computer vision. However, the real challenge is in enabling machine to carry out tasks that an average human does not have the skills for. It has enabled machine to do many human tasks. Computer Vision has been improved significantly in the past few decades.
